Motivation for the Experiment
The project's motivation is to improve the energy efficiency of IG 4.0 line designed to produce insulated glass. A digital twin will be created for that line and its gas-filling process will be optimized over a Machine Learning model. Insulated glass is used for energy efficiency in buildings and helps fewer greenhouse gas emissions (by better insulation). The factors affecting the energy efficiency of insulating glass are low-e glass, insulation, and gas fill. Therefore, the gas-filling process plays a crucial role in the energy efficiency level. Destructive tests for gas filling quality control are carried out manually in production. 0.5 % sampling rate is average for it.

IG 4.0 line at CMS
The cost and time of the quality test is 20 € and one hour. Since it is a destructive test, it is impossible to have 100 % quality control. And according to our analysis, the existing lines on the market have a 20-30 % defect production rate. That means that these products are not fulfilling their insulation performance of the buildings, however, as there is no strict control of buildings after commissioning, no authority is aware of that environmental impact. With this project, we make the defect product rate visible and aim to reduce it by 100% control of gas filling processes’ IIoT data over an AI model.
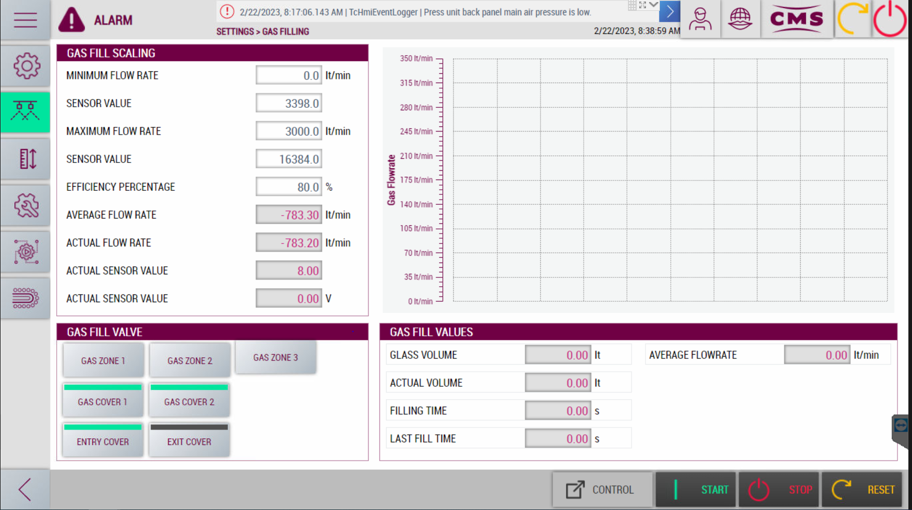
Gas Filling Process
Purpose of the Experiment
To optimize the gas filling process, a machine learning model will be used. The experiment partners will build a cycle analysis and anomaly detection tool to assure gas filling quality and show the output over a Digital Twin. High Performance Computing (HPC) will be used to speed up the process of building anomaly detection, classification and statistical analysis models (for model training) and achieve better performance. Meanwhile, the optimization tool (which will be supported by operator feedback and kind of things) will be developed during the project for it by the technical partner.
Partners are are planning to utilize the cloud/HPC platform that's because we can scale and adapt at speed and scale, accelerate innovation, drive business agility, streamline operations, and reduce costs. During the project process as software, the experiment will use Nodered, Docker, Digit Brain Platform, Fabmetrics (which is Simtera’s product), and Cloud platform (AWS).
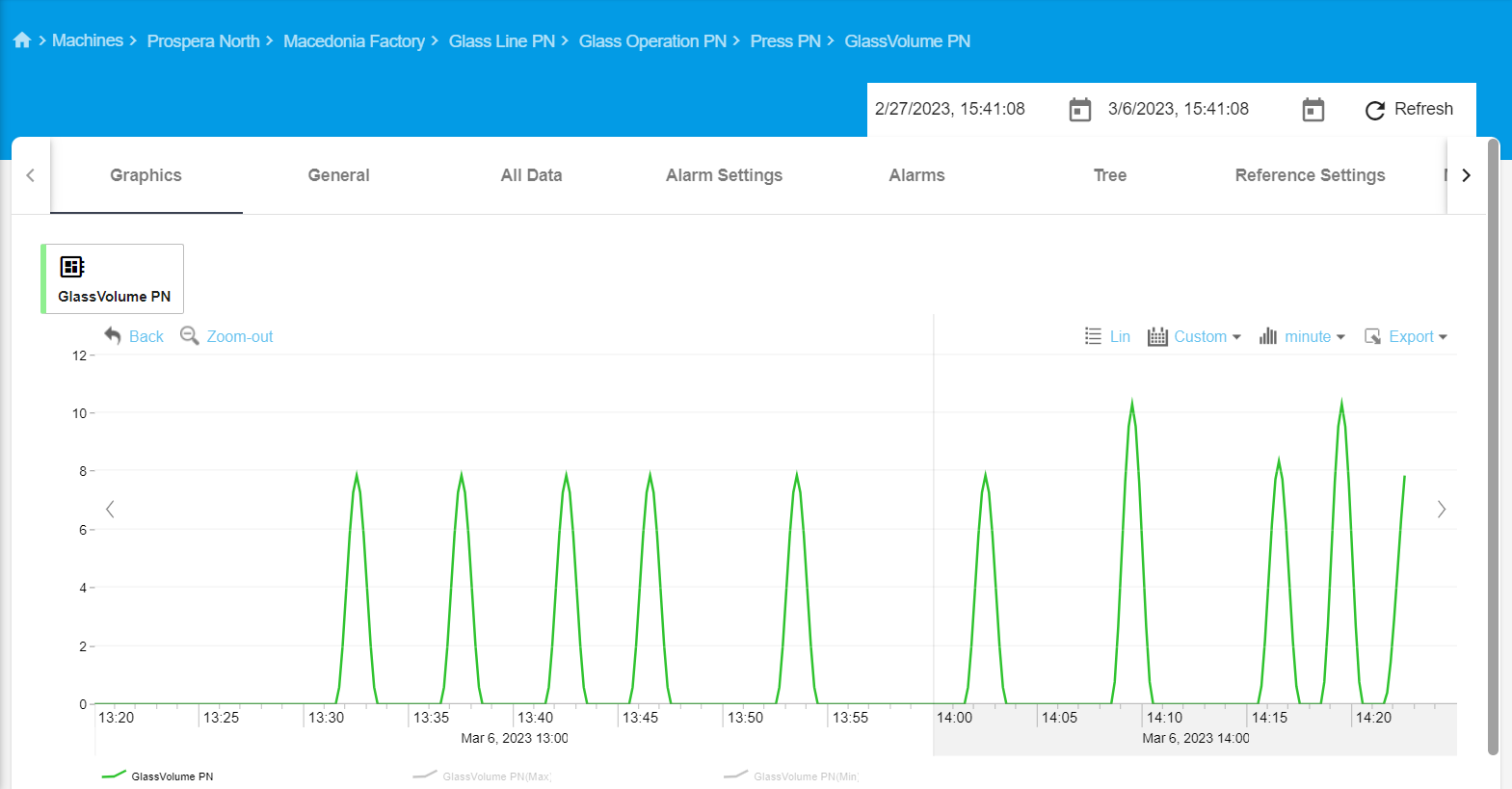
Glass Volume
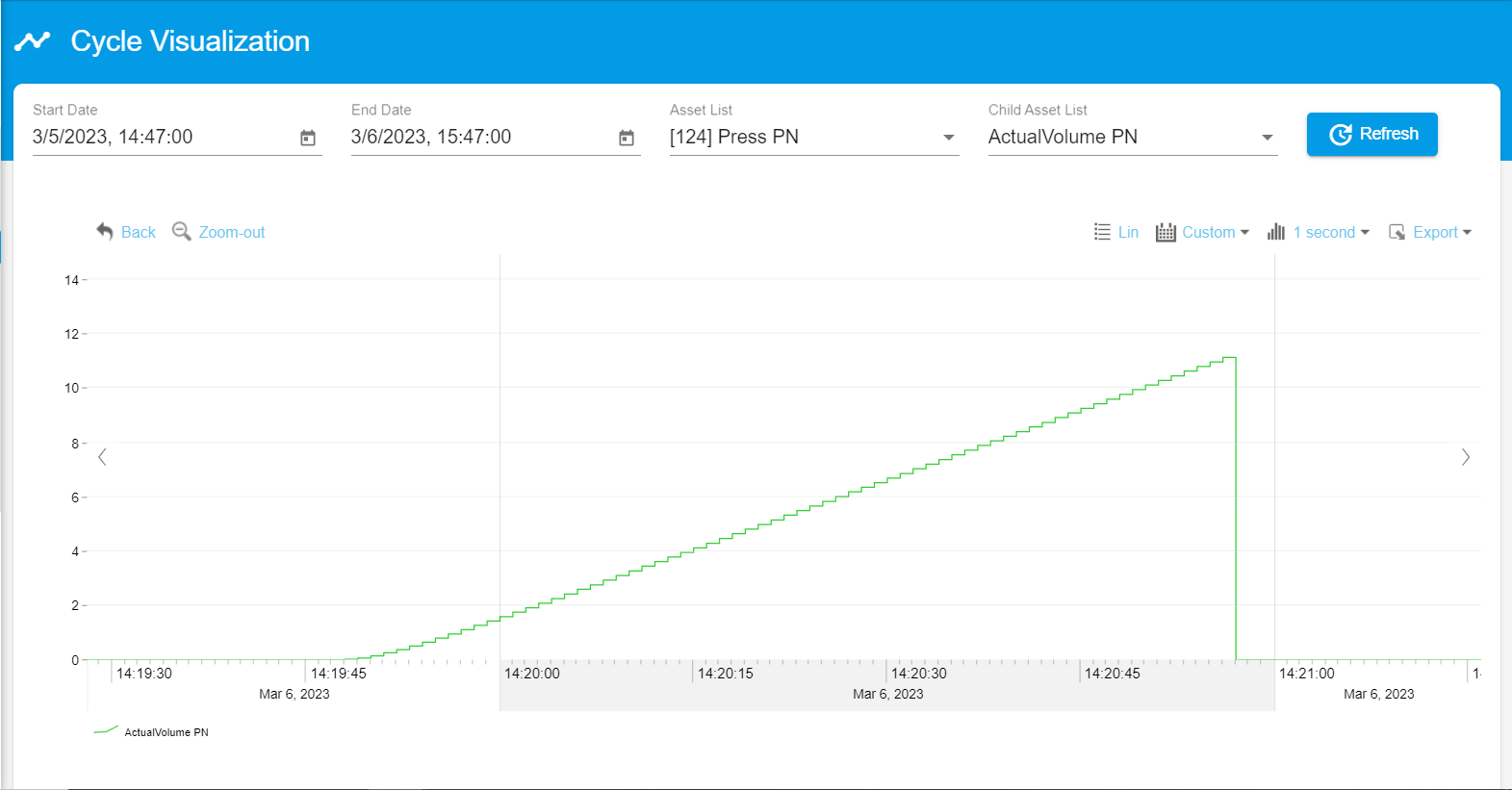
Actual Volume
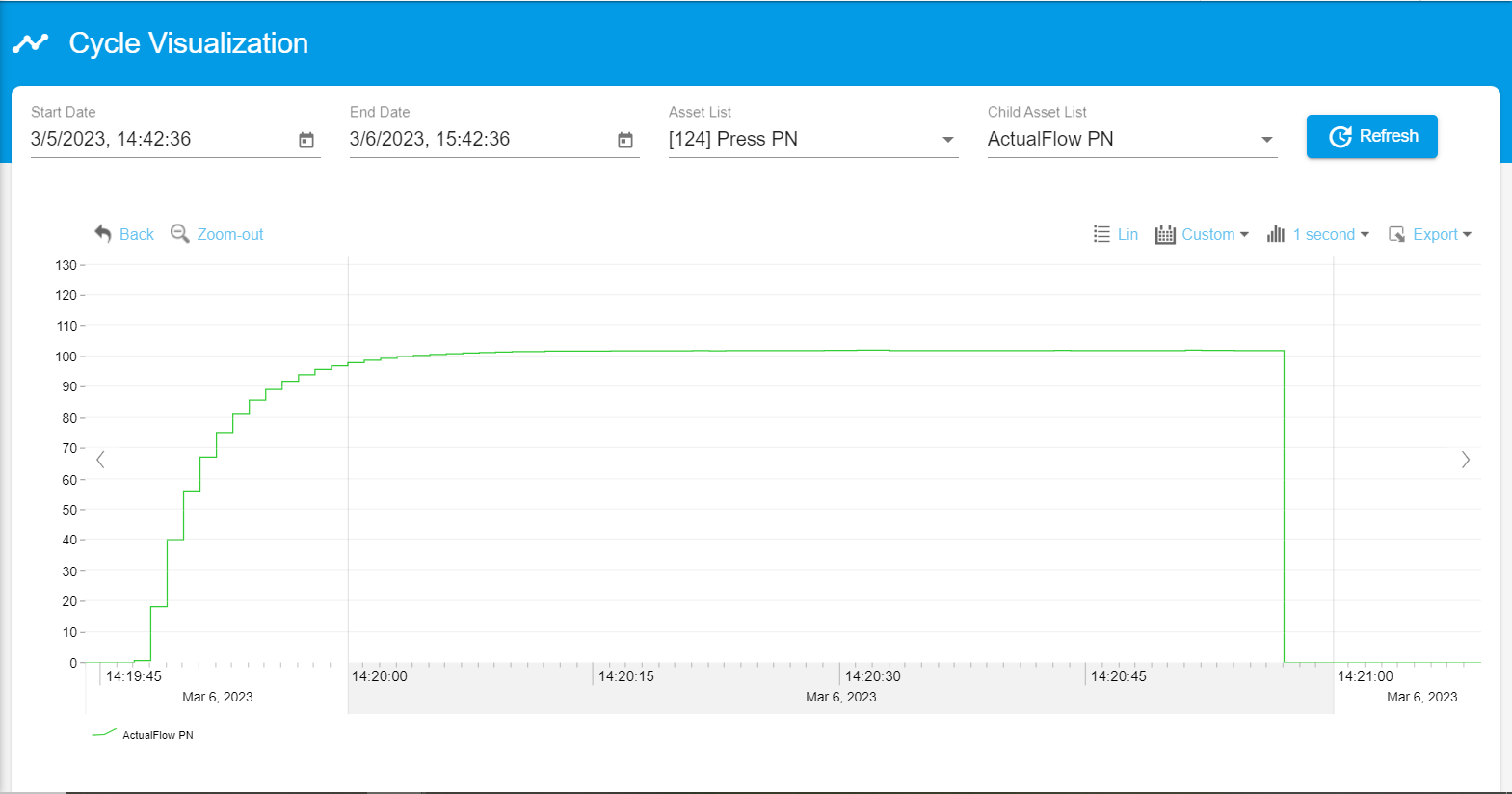
Actual Flow
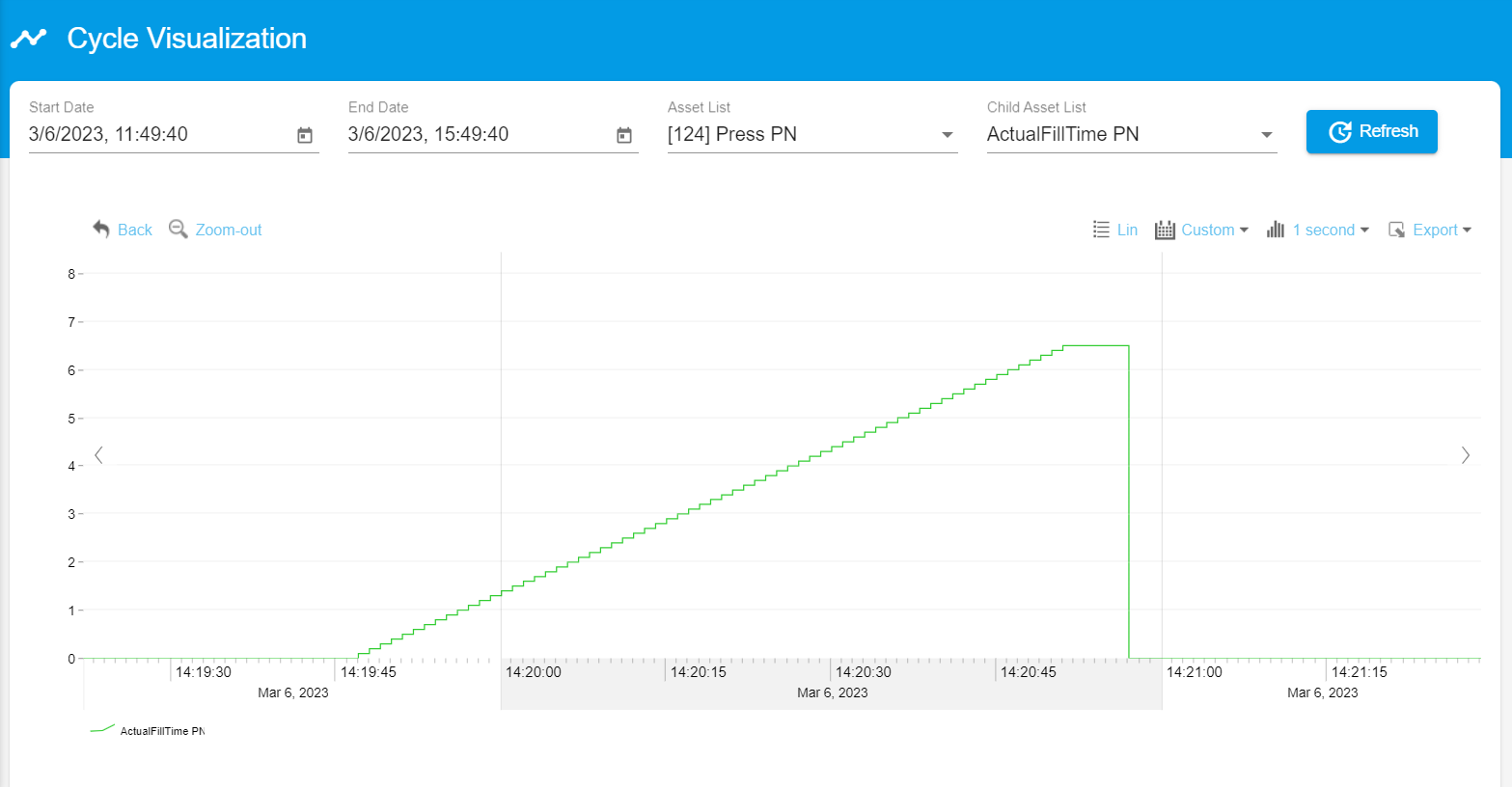
Actual Fill time
Technical Impact
With the topic of glass production CMS will bring a new industry for the diverse DIGITBrain portfolio. DTPQS4IG experiment unites an all turkish team (or almost - the Danish DIH DIGIT is supporting them) and therefore aims to increase the name regocnition for DIGITbrain technology and the DIGITbrain Digital Marketplace.
At the end of the experiment on the validation test phase, Simterra will do a few iterations for testing the models. They will produce different lots and manipulate affecting process parameters and sensors to obtain ‘glass that'd not filled enough’ and subsequently check the performance of the algorithm to detect these manipulated production lots. Detailed KPIs for that validation will be developed during the requirements analysis phase of the project.
Simtera is experienced in testing and validating cloud services and during the project, the Simtera team will give feedback to the DIGITbrain team with possible bugs and problems. By implementing their solution on the DIGITbrain Digital Agora, Simterra will contribute to the increase of application areas in the DIGITBrain portfolio and aims to convince their customers to use the solution on the platform. Further, Simtera will also add value by integrating DIGITBrain with native cloud services (AWS). Simtera has already performed a similar task by integrating the EFPF platform into AWS Lookout for Equipment service. In this project, AWS’ newly developed IoT Twin Maker service will be integrated into the DIGITBrain cloud solution.
Expected Economic Impact
The product to be developed this project will be the first Digital Service of CMS machinery. After this project, by CMS to manage this new product (including Digital Twin offerings) and manage next projects, they are planning to hire two new engineers. Further, with the marketing of the digital services, CMS will also hire ‘Sales Personnel’. Within 5 years, CMS plan to have a group of 5 engineers under the ‘digital services / digitalization’ business unit.
It is expected that 5 % of the Glass Machinery’s income will be generated from digital services within the upcoming 5 years. On that roadmap of 5 years, CMS’ plans € 10.000 income stream for 2023 from that service and projects alone. And a revenue of € 300.000 is projected from digital services by 2028.
Simtera plans to add a new intern to the team. In that sense it can create jobs as the project starts. However, with Digital Twin and extension Glass Industry customizations, that will correspond to 5 AI engineers,2 software developers and 2 salespersons within a period of 5 years.
As a digital servitisation platform for machine builders, on the big picture we are part of two markets:
- Manufacturing Analytics Market (AIoT) - est. to reach $ 102.2 billion by 2026 (IoT Analytics)
- Low Code Development Platform - $ 13.8 billion on 2021 (Gartner)
On industry specific (Machinery), BCG estimates within 5 years 5 % of their income will come from digital services. So, the total addressable market (TAM) is € 75 billion. Considering our focus industries (Plastics, Metal, Food + Glass) and regions (TR, DE), the serviceable obtainable market (SOM) will be € 1.2 billion by 2024. Considering we just started up on that market, their market share is insignificant. However, Simtera expects to reach € 20 million by 2025 which will correspond to 1,6 % of the SOM. When the glass industry is added to Simtera’s SOM, it is estimated that it can contribute an extra 5-10 % to its growth plans. A total frame of 5 years that can correspond to an extra revenue of € 8 million (Just 2028 € 2.5 million).
Project Partners:
CMS
is the end-user
in this experiment
Simtera
is the digital servization platform
(fabmetrics) provider
DIGIT
is the supporting DIH in the experiment