Motivation of the Experiment
Insoletwin will create a digital twin of the process of thermo-moulding within the manufacturing of shoe insoles in the factory of Arneplant, the EU leader in shoe insole manufacturing. The project will be based on the existing solution of Digitaltwin Solutions. The Digital Twin solution will be based in the data, model and algorithm tuple proposed by DigitBrain with the aim to use the Digital Agora in their exploitation. The large number of orders, added to the large amount of variety that an order can have, added to the numerous customizations that customers want for each of their orders makes our resource management impossible to manage by human beings. As a result, every day we have more than 21,000 different orders going around our warehouse and being processed by our machines. Achieving a perfect organization of resources and time has become a huge challenge that is collapsing one of our main processes.
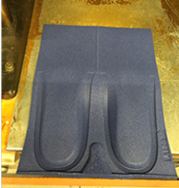
Purpose of the Experiment
The objective is to develop a scalable algorithm fed with information in real time that is capable of distributing orders in the machines, optimizing waiting time, electricity consumption and the availability of limited resources. All these variables and casuistics make it impossible for a conventional mathematical algorithm to find a favorable solution in a short time. So we need computing power and more sophisticated algorithms and techniques. In principle we are going to try to address the problem with genetic algorithms, they are a type of algorithms that can explore many search spaces in a short time and can address multifactorial problems.
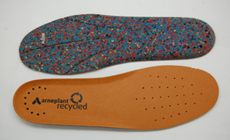
Technical Impact
After developing the solution, we will have a great impact at the organizational level, so we will not need so many resources for the management and organizational part and thus allocate more resources to what is really important, which is to scale and increase manufacturing. We will reduce the use of electricity, which is a great incentive in these times of energy crisis. We will optimize the use of space in our warehouses, since everything will be cleaner and orderlier and it will be easier to locate the assets since we will gain more speed in the manufacturing flow.
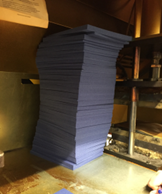
Expected Economic Impact
It is difficult to estimate the economic benefit in the short term, but the figures are very optimistic. We are going to be able to reduce the cost of this process in a very significant way. We estimate to reduce electricity losses by 5-10%, which today represents a large amount of money. Also by reducing manufacturing time we will be able to absorb many more customers, and with the same working time we will be able to service more orders, which means that we will grow and we will be able to generate more jobs.
Project Partners:
Arneplant SL
is the end-user
in this experiment
DigitalTwin Technology GmbH
is the software
provider
ITAINNOVA
is the Digital Innovation Hub with the role of experiment supervisor.